Random Sampling
Simple random sampling is a type of probability sampling in which the researcher randomly selects a subset of participants from a population. Each member of the population has an equal chance of being selected. Data is then collected from as large a percentage as possible of this random subset
Random sampling is a fundamental concept in statistics and research methodology. It refers to the process of selecting a subset of individuals, items, or data points from a larger population, where each member of the population has an equal chance of being chosen. Random sampling is used to ensure that the sample represents the population as accurately as possible, minimizing bias and increasing the generalizability of the results. This method is widely used across various disciplines, including social sciences, market research, healthcare studies, and quality control, to name a few.
In this detailed overview, we will discuss the importance of random sampling, its types, the steps involved in implementing it, and its advantages and limitations.
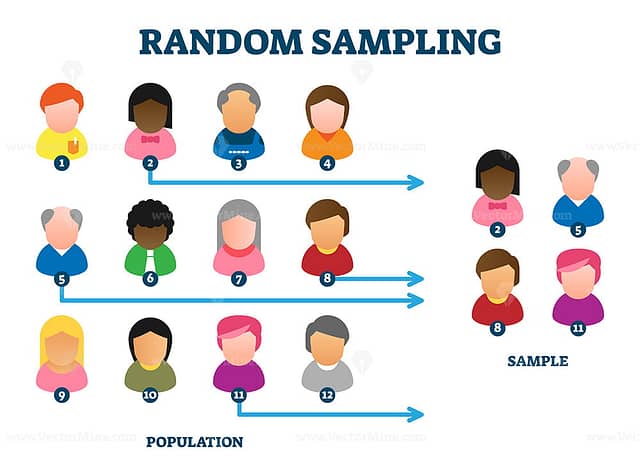
Importance of Random Sampling
Random sampling is crucial because it helps researchers make inferences about a population from a sample, reducing the potential for sampling bias. Bias occurs when certain members of the population have a higher chance of being selected than others, leading to a distorted representation of the entire population. Random sampling helps to prevent this issue by giving each individual or item an equal probability of being chosen.
The accuracy and fairness of random sampling enable researchers to draw more reliable conclusions from their studies, leading to valid and scientifically sound results. Moreover, random sampling is often a prerequisite for applying statistical methods and making predictions, as many inferential statistics, such as hypothesis testing and confidence intervals, assume that the sample is representative of the population.
Types of Random Sampling
There are several types of random sampling, each suited to different research designs and objectives. These methods include:
Simple Random Sampling
In simple random sampling, every member of the population has an equal chance of being selected. This method is one of the most straightforward forms of random sampling and ensures that the sample is unbiased. Simple random sampling can be done using random number generators, drawing names from a hat, or other random selection techniques.
Example: If you want to select 10 students from a class of 100, you can assign each student a number from 1 to 100 and use a random number generator to pick 10 numbers.
Systematic Sampling
Systematic sampling involves selecting every kth element from a population list. The starting point is chosen randomly, and after that, every kth member is included in the sample. Systematic sampling is often used when the population is already organized in a list or sequence.
Example: If you are selecting every 5th person from a list of 200 names, you would randomly choose a starting point, say number 3, and then select every 5th person after that (i.e., 3, 8, 13, 18, and so on).
Stratified Random Sampling
Stratified sampling involves dividing the population into subgroups or strata based on a shared characteristic, such as age, gender, income, or education level. Then, a random sample is taken from each stratum. This method is particularly useful when the population is heterogeneous, as it ensures that each subgroup is properly represented in the sample.
Example: In a survey about income levels, you might divide the population into strata based on income ranges (e.g., low, middle, and high income) and then randomly select individuals from each income group.
Cluster Sampling
Cluster sampling is used when it is difficult or expensive to create a list of all individuals in the population. The population is divided into clusters, usually based on geographic regions or other natural groupings, and then a random sample of clusters is selected. After selecting the clusters, every member of the chosen clusters is included in the sample. This method is useful when the population is spread out over a wide area.
Example: In a study of school performance, researchers might randomly select a few schools (clusters) and then survey all students in those selected schools.
Multistage Sampling
Multistage sampling is a combination of different sampling methods. For instance, a researcher may start with cluster sampling to select broad groups and then apply stratified or simple random sampling within those groups. This method is often used in large-scale surveys and complex research designs.
Example: In a national survey, a researcher might use cluster sampling to select regions, followed by stratified sampling to choose cities within those regions, and then simple random sampling to choose individuals from those cities.
Random Sampling in Public Health and Epidemiology
In public health and epidemiological research, random sampling is often used to study the distribution and determinants of health-related conditions across populations. Epidemiologists frequently use random sampling when conducting surveys or observational studies to estimate the prevalence of diseases, risk factors, or health behaviors. This method helps ensure that the sample is representative of the entire population, which is crucial for drawing accurate conclusions about public health trends and guiding policy decisions.
For example, in a national survey about smoking habits, a public health organization might use random sampling to select individuals from different regions, age groups, and socioeconomic statuses. This approach ensures that the data collected reflects the diversity of the population, enabling policymakers to understand the scope of smoking behaviors in the country and design targeted interventions.
Moreover, random sampling is critical when studying the spread of infectious diseases. Researchers conducting cohort studies on the effectiveness of a vaccine will randomly select participants from the population, helping ensure that the findings can be generalized to broader groups. Random sampling also reduces biases associated with self-selection, which can be a concern in studies where individuals volunteer to participate.
Random Sampling in Market Research
In market research, random sampling is essential for obtaining a reliable and unbiased representation of consumers’ preferences, behaviors, and attitudes toward products, services, or brands. Companies and organizations often use random sampling techniques to conduct surveys or focus groups to understand their target audience and make informed decisions about product development, advertising strategies, and customer satisfaction.
For instance, when launching a new product, a company may use random sampling to select consumers from different demographic segments (e.g., age, gender, location) to gather feedback on the product’s features, pricing, and overall appeal. This ensures that the sample reflects the diversity of the customer base, making the insights more accurate and actionable. Random sampling also helps to avoid the risk of bias introduced by selecting only loyal customers or certain groups that may not represent the broader market.
Additionally, random sampling in market research allows researchers to estimate the effectiveness of different marketing campaigns by comparing the responses of randomly selected participants who were exposed to different marketing strategies. The unbiased nature of random sampling is key in determining whether a particular approach works across a wide range of consumers or only among a specific subset.
Random Sampling in Quality Control and Manufacturing
In quality control (QC) and manufacturing processes, random sampling is frequently used to ensure that products meet the desired specifications and standards. Random sampling in QC involves selecting a subset of products from a production batch and testing them for defects, functionality, or consistency. The goal is to detect any issues with the production process and ensure that the final product is reliable and safe for consumers.
For example, in a factory producing electronic devices, a random sample of items from each production run may be selected to undergo rigorous testing for defects or malfunctions. By using random sampling, manufacturers can make inferences about the quality of the entire batch without needing to test every single product, saving both time and resources. If the sample reveals defects, corrective actions can be taken, such as adjusting machinery or refining the manufacturing process.
Furthermore, random sampling in quality control helps detect and correct problems early in the production process, ensuring that products maintain consistent quality standards. The technique is often paired with statistical process control (SPC) methods, where random samples are used to track the consistency of measurements (e.g., weight, dimensions, or strength) over time. This allows manufacturers to quickly identify deviations from the desired specifications and take corrective actions before large batches of defective products reach consumers.